Expo
view channel
view channel
view channel
view channel
view channel
view channel
view channel
view channel
view channel
Clinical Chem.Molecular DiagnosticsHematologyImmunologyMicrobiology
TechnologyIndustry
Events
- POC Biomedical Test Spins Water Droplet Using Sound Waves for Cancer Detection
- Highly Reliable Cell-Based Assay Enables Accurate Diagnosis of Endocrine Diseases
- New Blood Testing Method Detects Potent Opioids in Under Three Minutes
- Wireless Hepatitis B Test Kit Completes Screening and Data Collection in One Step
- Pain-Free, Low-Cost, Sensitive, Radiation-Free Device Detects Breast Cancer in Urine
- Ultrasound-Aided Blood Testing Detects Cancer Biomarkers from Cells
- New DNA Origami Technique to Advance Disease Diagnosis
- New Respiratory Syndromic Testing Panel Provides Fast and Accurate Results
- New Synthetic Biomarker Technology Differentiates Between Prior Zika and Dengue Infections
- Novel Biomarkers to Improve Diagnosis of Renal Cell Carcinoma Subtypes
- First 4-in-1 Nucleic Acid Test for Arbovirus Screening to Reduce Risk of Transfusion-Transmitted Infections
- POC Finger-Prick Blood Test Determines Risk of Neutropenic Sepsis in Patients Undergoing Chemotherapy
- First Affordable and Rapid Test for Beta Thalassemia Demonstrates 99% Diagnostic Accuracy
- Handheld White Blood Cell Tracker to Enable Rapid Testing For Infections
- Smart Palm-size Optofluidic Hematology Analyzer Enables POCT of Patients’ Blood Cells
- Diagnostic Blood Test for Cellular Rejection after Organ Transplant Could Replace Surgical Biopsies
- AI Tool Precisely Matches Cancer Drugs to Patients Using Information from Each Tumor Cell
- Genetic Testing Combined With Personalized Drug Screening On Tumor Samples to Revolutionize Cancer Treatment
- Testing Method Could Help More Patients Receive Right Cancer Treatment
- Groundbreaking Test Monitors Radiation Therapy Toxicity in Cancer Patients
- Automated Sepsis Test System Enables Rapid Diagnosis for Patients with Severe Bloodstream Infections
- Enhanced Rapid Syndromic Molecular Diagnostic Solution Detects Broad Range of Infectious Diseases
- Clinical Decision Support Software a Game-Changer in Antimicrobial Resistance Battle
- New CE-Marked Hepatitis Assays to Help Diagnose Infections Earlier
- 1 Hour, Direct-From-Blood Multiplex PCR Test Identifies 95% of Sepsis-Causing Pathogens
- DNA Biosensor Enables Early Diagnosis of Cervical Cancer
- Self-Heating Microfluidic Devices Can Detect Diseases in Tiny Blood or Fluid Samples
- Breakthrough in Diagnostic Technology Could Make On-The-Spot Testing Widely Accessible
- First of Its Kind Technology Detects Glucose in Human Saliva
- Electrochemical Device Identifies People at Higher Risk for Osteoporosis Using Single Blood Drop
- Beckman Coulter and MeMed Expand Host Immune Response Diagnostics Partnership
- Thermo Fisher and Bio-Techne Enter Into Strategic Distribution Agreement for Europe
- ECCMID Congress Name Changes to ESCMID Global
- Bosch and Randox Partner to Make Strategic Investment in Vivalytic Analysis Platform
- Siemens to Close Fast Track Diagnostics Business
- Gene Panel Predicts Disease Progession for Patients with B-cell Lymphoma
- New Method Simplifies Preparation of Tumor Genomic DNA Libraries
- New Tool Developed for Diagnosis of Chronic HBV Infection
- Panel of Genetic Loci Accurately Predicts Risk of Developing Gout
- Disrupted TGFB Signaling Linked to Increased Cancer-Related Bacteria
- HPV Self-Collection Solution Improves Access to Cervical Cancer Testing
- Hyperspectral Dark-Field Microscopy Enables Rapid and Accurate Identification of Cancerous Tissues
- AI Advancements Enable Leap into 3D Pathology
- New Blood Test Device Modeled on Leeches to Help Diagnose Malaria
- Robotic Blood Drawing Device to Revolutionize Sample Collection for Diagnostic Testing
Expo
view channel
view channel
view channel
view channel
view channel
view channel
view channel
view channel
view channel
Clinical Chem.Molecular DiagnosticsHematologyImmunologyMicrobiology
TechnologyIndustry
Events
Advertise with Us
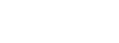
- POC Biomedical Test Spins Water Droplet Using Sound Waves for Cancer Detection
- Highly Reliable Cell-Based Assay Enables Accurate Diagnosis of Endocrine Diseases
- New Blood Testing Method Detects Potent Opioids in Under Three Minutes
- Wireless Hepatitis B Test Kit Completes Screening and Data Collection in One Step
- Pain-Free, Low-Cost, Sensitive, Radiation-Free Device Detects Breast Cancer in Urine
- Ultrasound-Aided Blood Testing Detects Cancer Biomarkers from Cells
- New DNA Origami Technique to Advance Disease Diagnosis
- New Respiratory Syndromic Testing Panel Provides Fast and Accurate Results
- New Synthetic Biomarker Technology Differentiates Between Prior Zika and Dengue Infections
- Novel Biomarkers to Improve Diagnosis of Renal Cell Carcinoma Subtypes
- First 4-in-1 Nucleic Acid Test for Arbovirus Screening to Reduce Risk of Transfusion-Transmitted Infections
- POC Finger-Prick Blood Test Determines Risk of Neutropenic Sepsis in Patients Undergoing Chemotherapy
- First Affordable and Rapid Test for Beta Thalassemia Demonstrates 99% Diagnostic Accuracy
- Handheld White Blood Cell Tracker to Enable Rapid Testing For Infections
- Smart Palm-size Optofluidic Hematology Analyzer Enables POCT of Patients’ Blood Cells
- Diagnostic Blood Test for Cellular Rejection after Organ Transplant Could Replace Surgical Biopsies
- AI Tool Precisely Matches Cancer Drugs to Patients Using Information from Each Tumor Cell
- Genetic Testing Combined With Personalized Drug Screening On Tumor Samples to Revolutionize Cancer Treatment
- Testing Method Could Help More Patients Receive Right Cancer Treatment
- Groundbreaking Test Monitors Radiation Therapy Toxicity in Cancer Patients
- Automated Sepsis Test System Enables Rapid Diagnosis for Patients with Severe Bloodstream Infections
- Enhanced Rapid Syndromic Molecular Diagnostic Solution Detects Broad Range of Infectious Diseases
- Clinical Decision Support Software a Game-Changer in Antimicrobial Resistance Battle
- New CE-Marked Hepatitis Assays to Help Diagnose Infections Earlier
- 1 Hour, Direct-From-Blood Multiplex PCR Test Identifies 95% of Sepsis-Causing Pathogens
- DNA Biosensor Enables Early Diagnosis of Cervical Cancer
- Self-Heating Microfluidic Devices Can Detect Diseases in Tiny Blood or Fluid Samples
- Breakthrough in Diagnostic Technology Could Make On-The-Spot Testing Widely Accessible
- First of Its Kind Technology Detects Glucose in Human Saliva
- Electrochemical Device Identifies People at Higher Risk for Osteoporosis Using Single Blood Drop
- Beckman Coulter and MeMed Expand Host Immune Response Diagnostics Partnership
- Thermo Fisher and Bio-Techne Enter Into Strategic Distribution Agreement for Europe
- ECCMID Congress Name Changes to ESCMID Global
- Bosch and Randox Partner to Make Strategic Investment in Vivalytic Analysis Platform
- Siemens to Close Fast Track Diagnostics Business
- Gene Panel Predicts Disease Progession for Patients with B-cell Lymphoma
- New Method Simplifies Preparation of Tumor Genomic DNA Libraries
- New Tool Developed for Diagnosis of Chronic HBV Infection
- Panel of Genetic Loci Accurately Predicts Risk of Developing Gout
- Disrupted TGFB Signaling Linked to Increased Cancer-Related Bacteria
- HPV Self-Collection Solution Improves Access to Cervical Cancer Testing
- Hyperspectral Dark-Field Microscopy Enables Rapid and Accurate Identification of Cancerous Tissues
- AI Advancements Enable Leap into 3D Pathology
- New Blood Test Device Modeled on Leeches to Help Diagnose Malaria
- Robotic Blood Drawing Device to Revolutionize Sample Collection for Diagnostic Testing