Expo
view channel
view channel
view channel
view channel
view channel
view channel
view channel
view channel
view channel
Clinical Chem.Molecular DiagnosticsHematologyImmunologyMicrobiology
TechnologyIndustry
Events
- Biosensing Technology Breakthrough Paves Way for New Methods of Early Disease Detection
- New Saliva Test Rapidly Identifies Paracetamol Overdose
- POC Saliva Testing Device Predicts Heart Failure in 15 Minutes
- Screening Tool Detects Multiple Health Conditions from Single Blood Drop
- Integrated Chemistry and Immunoassay Analyzer with Extensive Assay Menu Offers Flexibility, Scalability and Data Commutability
- Automated Free Testosterone Test Advances Diagnostic Capabilities for Androgen Disorders
- First Of Its Kind Blood Test Accurately Predicts Preterm Birth Risk
- Simple Blood Test Diagnoses Asthma and Determines Severity
- “TwinDemic" Diagnostic System Rapidly and Simultaneously Detects SARS-CoV-2 and Influenza A Virus
- Experimental Blood Test Could Catch Pancreatic Cancer More Quickly in High-Risk People
- Streamlined Approach to Testing for Heparin-Induced Thrombocytopenia Improves Diagnostic Accuracy
- POC Hemostasis System Could Help Prevent Maternal Deaths
- New Test Assesses Oxygen Delivering Ability of Red Blood Cells by Measuring Their Shape
- Personalized CBC Testing Could Help Diagnose Early-Stage Diseases in Healthy Individuals
- Non-Invasive Test Solution Determines Fetal RhD Status from Maternal Plasma
- AI Tool Uses Routine Blood Tests to Predict Immunotherapy Response for Various Cancers
- Blood Test Can Predict How Long Vaccine Immunity Will Last
- Microfluidic Chip-Based Device to Measure Viral Immunity
- Simple Blood Test Could Detect Drug Resistance in Ovarian Cancer Patients
- Advanced Imaging Method Maps Immune Cell Connections to Predict Cancer Patients Survival
- POC PCR Test Rapidly Detects Bacterial Meningitis Directly at Point of Sample Collection
- Genetic Testing Could Improve Treatment for Virulent Multidrug-Resistant Fungus Candida Auris
- Ultra-Rapid AST System Provides Critical Results for Sepsis Patients
- High-Throughput AST System Uses Microchip Technology to Rapidly Analyze Bacterial Samples
- Syndromic PCR Test Rapidly and Accurately Identifies Pathogens in Patients with Tropical Fever Infections
- FET-Based Sensors Pave Way for Portable Diagnostic Devices Capable of Detecting Multiple Diseases
- Paper-Based Biosensor System to Detect Glucose Using Sweat Could Revolutionize Diabetes Management
- First AI-Powered Blood Test Identifies Patients in Earliest Stage of Breast Cancer
- Optical Biosensor Rapidly Detects Monkeypox Virus at Point of Care
- Nanomaterial-Based Diagnostic Technology Accurately Monitors Drug Therapy in Epilepsy Patients
- Danaher Partners with Healthcare AI Company Innovaccer on Novel Digital and Diagnostic Solutions
- OraSure Technologies Acquires Sherlock Biosciences
- Worldwide Initiative Aims to Develop Globally Generalizable Blood-Based Tests for Alzheimer’s Disease
- Hologic Enters into Agreement with CDC for H5N1 Bird Flu Test Development
- Quanterix Acquires Magnetic Bead Tech Firm Emission
- Gene Panel Predicts Disease Progession for Patients with B-cell Lymphoma
- New Method Simplifies Preparation of Tumor Genomic DNA Libraries
- New Tool Developed for Diagnosis of Chronic HBV Infection
- Panel of Genetic Loci Accurately Predicts Risk of Developing Gout
- Disrupted TGFB Signaling Linked to Increased Cancer-Related Bacteria
- New Technique Predicts Tumor’s Responsiveness to Breast Cancer Treatment
- AI Tool Combines Data from Medical Images with Text to Predict Cancer Prognoses
- New Self-Collection Medical Test Makes HPV Screening Easier for Patients
- Biolasers Light Up Circulating Tumor Cells in Bloodstream of Cancer Patients
- Mini-Tumors Cultivated from Circulating Cancer Cells to Help Develop Targeted Therapies
Expo
view channel
view channel
view channel
view channel
view channel
view channel
view channel
view channel
view channel
Clinical Chem.Molecular DiagnosticsHematologyImmunologyMicrobiology
TechnologyIndustry
Events
Advertise with Us
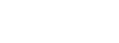
- Biosensing Technology Breakthrough Paves Way for New Methods of Early Disease Detection
- New Saliva Test Rapidly Identifies Paracetamol Overdose
- POC Saliva Testing Device Predicts Heart Failure in 15 Minutes
- Screening Tool Detects Multiple Health Conditions from Single Blood Drop
- Integrated Chemistry and Immunoassay Analyzer with Extensive Assay Menu Offers Flexibility, Scalability and Data Commutability
- Automated Free Testosterone Test Advances Diagnostic Capabilities for Androgen Disorders
- First Of Its Kind Blood Test Accurately Predicts Preterm Birth Risk
- Simple Blood Test Diagnoses Asthma and Determines Severity
- “TwinDemic" Diagnostic System Rapidly and Simultaneously Detects SARS-CoV-2 and Influenza A Virus
- Experimental Blood Test Could Catch Pancreatic Cancer More Quickly in High-Risk People
- Streamlined Approach to Testing for Heparin-Induced Thrombocytopenia Improves Diagnostic Accuracy
- POC Hemostasis System Could Help Prevent Maternal Deaths
- New Test Assesses Oxygen Delivering Ability of Red Blood Cells by Measuring Their Shape
- Personalized CBC Testing Could Help Diagnose Early-Stage Diseases in Healthy Individuals
- Non-Invasive Test Solution Determines Fetal RhD Status from Maternal Plasma
- AI Tool Uses Routine Blood Tests to Predict Immunotherapy Response for Various Cancers
- Blood Test Can Predict How Long Vaccine Immunity Will Last
- Microfluidic Chip-Based Device to Measure Viral Immunity
- Simple Blood Test Could Detect Drug Resistance in Ovarian Cancer Patients
- Advanced Imaging Method Maps Immune Cell Connections to Predict Cancer Patients Survival
- POC PCR Test Rapidly Detects Bacterial Meningitis Directly at Point of Sample Collection
- Genetic Testing Could Improve Treatment for Virulent Multidrug-Resistant Fungus Candida Auris
- Ultra-Rapid AST System Provides Critical Results for Sepsis Patients
- High-Throughput AST System Uses Microchip Technology to Rapidly Analyze Bacterial Samples
- Syndromic PCR Test Rapidly and Accurately Identifies Pathogens in Patients with Tropical Fever Infections
- FET-Based Sensors Pave Way for Portable Diagnostic Devices Capable of Detecting Multiple Diseases
- Paper-Based Biosensor System to Detect Glucose Using Sweat Could Revolutionize Diabetes Management
- First AI-Powered Blood Test Identifies Patients in Earliest Stage of Breast Cancer
- Optical Biosensor Rapidly Detects Monkeypox Virus at Point of Care
- Nanomaterial-Based Diagnostic Technology Accurately Monitors Drug Therapy in Epilepsy Patients
- Danaher Partners with Healthcare AI Company Innovaccer on Novel Digital and Diagnostic Solutions
- OraSure Technologies Acquires Sherlock Biosciences
- Worldwide Initiative Aims to Develop Globally Generalizable Blood-Based Tests for Alzheimer’s Disease
- Hologic Enters into Agreement with CDC for H5N1 Bird Flu Test Development
- Quanterix Acquires Magnetic Bead Tech Firm Emission
- Gene Panel Predicts Disease Progession for Patients with B-cell Lymphoma
- New Method Simplifies Preparation of Tumor Genomic DNA Libraries
- New Tool Developed for Diagnosis of Chronic HBV Infection
- Panel of Genetic Loci Accurately Predicts Risk of Developing Gout
- Disrupted TGFB Signaling Linked to Increased Cancer-Related Bacteria
- New Technique Predicts Tumor’s Responsiveness to Breast Cancer Treatment
- AI Tool Combines Data from Medical Images with Text to Predict Cancer Prognoses
- New Self-Collection Medical Test Makes HPV Screening Easier for Patients
- Biolasers Light Up Circulating Tumor Cells in Bloodstream of Cancer Patients
- Mini-Tumors Cultivated from Circulating Cancer Cells to Help Develop Targeted Therapies