Expo
view channel
view channel
view channel
view channel
view channel
view channel
view channel
view channel
view channel
Clinical Chem.Molecular DiagnosticsHematologyImmunology
PathologyTechnologyIndustry
Events
- Biosensing Technology Breakthrough Paves Way for New Methods of Early Disease Detection
- New Saliva Test Rapidly Identifies Paracetamol Overdose
- POC Saliva Testing Device Predicts Heart Failure in 15 Minutes
- Screening Tool Detects Multiple Health Conditions from Single Blood Drop
- Integrated Chemistry and Immunoassay Analyzer with Extensive Assay Menu Offers Flexibility, Scalability and Data Commutability
- Blood Test Accurately Detects Circulating Tumor-Related Mutations
- New Molecular Tests Diagnose STIs at POC
- New Protein Biomarkers to Improve Diagnostic Tools for Colorectal Cancer
- Robust Microbiome Signatures Enable More Precise Diagnoses of Nonalcoholic Fatty Liver Disease
- Urine-Based Bladder Cancer Diagnostic Kit to Reduce Need for Unnecessary Cystoscopies
- Streamlined Approach to Testing for Heparin-Induced Thrombocytopenia Improves Diagnostic Accuracy
- POC Hemostasis System Could Help Prevent Maternal Deaths
- New Test Assesses Oxygen Delivering Ability of Red Blood Cells by Measuring Their Shape
- Personalized CBC Testing Could Help Diagnose Early-Stage Diseases in Healthy Individuals
- Non-Invasive Test Solution Determines Fetal RhD Status from Maternal Plasma
- New Discovery in Blood Immune Cells Paves Way for Parkinson's Disease Diagnostic Test
- AI Tool Uses Routine Blood Tests to Predict Immunotherapy Response for Various Cancers
- Blood Test Can Predict How Long Vaccine Immunity Will Last
- Microfluidic Chip-Based Device to Measure Viral Immunity
- Simple Blood Test Could Detect Drug Resistance in Ovarian Cancer Patients
- CRISPR-TB Blood Test to Enable Early Disease Diagnosis and Public Screening
- Syndromic Panel Provides Fast Answers for Outpatient Diagnosis of Gastrointestinal Conditions
- Culture-Free Platform Rapidly Identifies Blood Stream Infections
- POC PCR Test Rapidly Detects Bacterial Meningitis Directly at Point of Sample Collection
- Genetic Testing Could Improve Treatment for Virulent Multidrug-Resistant Fungus Candida Auris
- New Lens Method Analyzes Tears for Early Disease Detection
- FET-Based Sensors Pave Way for Portable Diagnostic Devices Capable of Detecting Multiple Diseases
- Paper-Based Biosensor System to Detect Glucose Using Sweat Could Revolutionize Diabetes Management
- First AI-Powered Blood Test Identifies Patients in Earliest Stage of Breast Cancer
- Optical Biosensor Rapidly Detects Monkeypox Virus at Point of Care
- Medix Biochemica Acquires German Immunoassay Solutions Developer Candor Bioscience
- bioMérieux Acquires Norwegian Immunoassay Start-Up SpinChip Diagnostics
- Danaher Partners with Healthcare AI Company Innovaccer on Novel Digital and Diagnostic Solutions
- OraSure Technologies Acquires Sherlock Biosciences
- Worldwide Initiative Aims to Develop Globally Generalizable Blood-Based Tests for Alzheimer’s Disease
- Gene Panel Predicts Disease Progession for Patients with B-cell Lymphoma
- New Method Simplifies Preparation of Tumor Genomic DNA Libraries
- New Tool Developed for Diagnosis of Chronic HBV Infection
- Panel of Genetic Loci Accurately Predicts Risk of Developing Gout
- Disrupted TGFB Signaling Linked to Increased Cancer-Related Bacteria
- Biomarker Algorithm Enables Noninvasive Detection of Barrett’s Esophagus and Esophageal Cancer
- AI Tool Analyzes 30K Data Points Per Medical Imaging Pixel in Cancer Search
- New Technique Predicts Tumor’s Responsiveness to Breast Cancer Treatment
- AI Tool Combines Data from Medical Images with Text to Predict Cancer Prognoses
- New Self-Collection Medical Test Makes HPV Screening Easier for Patients
Expo
view channel
view channel
view channel
view channel
view channel
view channel
view channel
view channel
view channel
Clinical Chem.Molecular DiagnosticsHematologyImmunology
PathologyTechnologyIndustry
Events
Advertise with Us
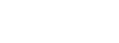
- Biosensing Technology Breakthrough Paves Way for New Methods of Early Disease Detection
- New Saliva Test Rapidly Identifies Paracetamol Overdose
- POC Saliva Testing Device Predicts Heart Failure in 15 Minutes
- Screening Tool Detects Multiple Health Conditions from Single Blood Drop
- Integrated Chemistry and Immunoassay Analyzer with Extensive Assay Menu Offers Flexibility, Scalability and Data Commutability
- Blood Test Accurately Detects Circulating Tumor-Related Mutations
- New Molecular Tests Diagnose STIs at POC
- New Protein Biomarkers to Improve Diagnostic Tools for Colorectal Cancer
- Robust Microbiome Signatures Enable More Precise Diagnoses of Nonalcoholic Fatty Liver Disease
- Urine-Based Bladder Cancer Diagnostic Kit to Reduce Need for Unnecessary Cystoscopies
- Streamlined Approach to Testing for Heparin-Induced Thrombocytopenia Improves Diagnostic Accuracy
- POC Hemostasis System Could Help Prevent Maternal Deaths
- New Test Assesses Oxygen Delivering Ability of Red Blood Cells by Measuring Their Shape
- Personalized CBC Testing Could Help Diagnose Early-Stage Diseases in Healthy Individuals
- Non-Invasive Test Solution Determines Fetal RhD Status from Maternal Plasma
- New Discovery in Blood Immune Cells Paves Way for Parkinson's Disease Diagnostic Test
- AI Tool Uses Routine Blood Tests to Predict Immunotherapy Response for Various Cancers
- Blood Test Can Predict How Long Vaccine Immunity Will Last
- Microfluidic Chip-Based Device to Measure Viral Immunity
- Simple Blood Test Could Detect Drug Resistance in Ovarian Cancer Patients
- CRISPR-TB Blood Test to Enable Early Disease Diagnosis and Public Screening
- Syndromic Panel Provides Fast Answers for Outpatient Diagnosis of Gastrointestinal Conditions
- Culture-Free Platform Rapidly Identifies Blood Stream Infections
- POC PCR Test Rapidly Detects Bacterial Meningitis Directly at Point of Sample Collection
- Genetic Testing Could Improve Treatment for Virulent Multidrug-Resistant Fungus Candida Auris
- New Lens Method Analyzes Tears for Early Disease Detection
- FET-Based Sensors Pave Way for Portable Diagnostic Devices Capable of Detecting Multiple Diseases
- Paper-Based Biosensor System to Detect Glucose Using Sweat Could Revolutionize Diabetes Management
- First AI-Powered Blood Test Identifies Patients in Earliest Stage of Breast Cancer
- Optical Biosensor Rapidly Detects Monkeypox Virus at Point of Care
- Medix Biochemica Acquires German Immunoassay Solutions Developer Candor Bioscience
- bioMérieux Acquires Norwegian Immunoassay Start-Up SpinChip Diagnostics
- Danaher Partners with Healthcare AI Company Innovaccer on Novel Digital and Diagnostic Solutions
- OraSure Technologies Acquires Sherlock Biosciences
- Worldwide Initiative Aims to Develop Globally Generalizable Blood-Based Tests for Alzheimer’s Disease
- Gene Panel Predicts Disease Progession for Patients with B-cell Lymphoma
- New Method Simplifies Preparation of Tumor Genomic DNA Libraries
- New Tool Developed for Diagnosis of Chronic HBV Infection
- Panel of Genetic Loci Accurately Predicts Risk of Developing Gout
- Disrupted TGFB Signaling Linked to Increased Cancer-Related Bacteria
- Biomarker Algorithm Enables Noninvasive Detection of Barrett’s Esophagus and Esophageal Cancer
- AI Tool Analyzes 30K Data Points Per Medical Imaging Pixel in Cancer Search
- New Technique Predicts Tumor’s Responsiveness to Breast Cancer Treatment
- AI Tool Combines Data from Medical Images with Text to Predict Cancer Prognoses
- New Self-Collection Medical Test Makes HPV Screening Easier for Patients