Expo
view channel
view channel
view channel
view channel
view channel
view channel
view channel
view channel
view channel
Clinical Chem.Molecular DiagnosticsHematologyImmunologyMicrobiology
TechnologyIndustry
Events
- Paper-Based Device Boosts HIV Test Accuracy from Dried Blood Samples
- AI-Powered Raman Spectroscopy Method Enables Rapid Drug Detection in Blood
- Novel LC-MS/MS Assay Detects Low Creatinine in Sweat and Saliva
- Biosensing Technology Breakthrough Paves Way for New Methods of Early Disease Detection
- New Saliva Test Rapidly Identifies Paracetamol Overdose
- Blood Test Rules Out Future Dementia Risk
- D-Dimer Testing Can Identify Patients at Higher Risk of Pulmonary Embolism
- New Biomarkers to Improve Early Detection and Monitoring of Kidney Injury
- Chemiluminescence Immunoassays Support Diagnosis of Alzheimer’s Disease
- Blood Test Identifies Multiple Biomarkers for Rapid Diagnosis of Spinal Cord Injury
- Non-Invasive Prenatal Test for Fetal RhD Status Demonstrates 100% Accuracy
- WBC Count Could Predict Severity of COVID-19 Symptoms
- New Platelet Counting Technology to Help Labs Prevent Diagnosis Errors
- Streamlined Approach to Testing for Heparin-Induced Thrombocytopenia Improves Diagnostic Accuracy
- POC Hemostasis System Could Help Prevent Maternal Deaths
- Machine Learning-Enabled Blood Test Predicts Immunotherapy Response in Lymphoma Patients
- Post-Treatment Blood Test Could Inform Future Cancer Therapy Decisions
- Cerebrospinal Fluid Test Predicts Dangerous Side Effect of Cancer Treatment
- New Test Measures Preterm Infant Immunity Using Only Two Drops of Blood
- Simple Blood Test Could Help Choose Better Treatments for Patients with Recurrent Endometrial Cancer
- Breakthrough Diagnostic Technology Identifies Bacterial Infections with Almost 100% Accuracy within Three Hours
- Innovative ID/AST System to Help Diagnose Infectious Diseases and Combat AMR
- Gastrointestinal Panel Delivers Rapid Detection of Five Common Bacterial Pathogens for Outpatient Use
- Rapid PCR Testing in ICU Improves Antibiotic Stewardship
- Unique Genetic Signature Predicts Drug Resistance in Bacteria
- Smartphones Could Diagnose Diseases Using Infrared Scans
- Novel Sensor Technology to Enable Early Diagnoses of Metabolic and Cardiovascular Disorders
- 3D Printing Breakthrough Enables Large Scale Development of Tiny Microfluidic Devices
- POC Paper-Based Sensor Platform to Transform Cardiac Diagnostics
- Study Explores Impact of POC Testing on Future of Diagnostics
- New Collaboration to Advance Microbial Identification for Infectious Disease Diagnostics
- Tecan Acquires ELISA Immunoassay Assets from Revvity's Cisbio Bioassays
- Leica Biosystems and Bio-Techne Expand Spatial Multiomic Collaboration
- Philips and Ibex Expand Partnership to Enhance AI-Enabled Pathology Workflows
- Grifols and Inpeco Partner to Deliver Transfusion Medicine ‘Lab of The Future’
- Gene Panel Predicts Disease Progession for Patients with B-cell Lymphoma
- New Method Simplifies Preparation of Tumor Genomic DNA Libraries
- New Tool Developed for Diagnosis of Chronic HBV Infection
- Panel of Genetic Loci Accurately Predicts Risk of Developing Gout
- Disrupted TGFB Signaling Linked to Increased Cancer-Related Bacteria
- New Laser-Based Method to Accelerate Cancer Diagnosis
- New AI Model Predicts Gene Variants’ Effects on Specific Diseases
- Powerful AI Tool Diagnoses Coeliac Disease from Biopsy Images with Over 97% Accuracy
- Pre-Analytical Conditions Influence Cell-Free MicroRNA Stability in Blood Plasma Samples
- 3D Cell Culture System Could Revolutionize Cancer Diagnostics
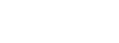
- Paper-Based Device Boosts HIV Test Accuracy from Dried Blood Samples
- AI-Powered Raman Spectroscopy Method Enables Rapid Drug Detection in Blood
- Novel LC-MS/MS Assay Detects Low Creatinine in Sweat and Saliva
- Biosensing Technology Breakthrough Paves Way for New Methods of Early Disease Detection
- New Saliva Test Rapidly Identifies Paracetamol Overdose
- Blood Test Rules Out Future Dementia Risk
- D-Dimer Testing Can Identify Patients at Higher Risk of Pulmonary Embolism
- New Biomarkers to Improve Early Detection and Monitoring of Kidney Injury
- Chemiluminescence Immunoassays Support Diagnosis of Alzheimer’s Disease
- Blood Test Identifies Multiple Biomarkers for Rapid Diagnosis of Spinal Cord Injury
- Non-Invasive Prenatal Test for Fetal RhD Status Demonstrates 100% Accuracy
- WBC Count Could Predict Severity of COVID-19 Symptoms
- New Platelet Counting Technology to Help Labs Prevent Diagnosis Errors
- Streamlined Approach to Testing for Heparin-Induced Thrombocytopenia Improves Diagnostic Accuracy
- POC Hemostasis System Could Help Prevent Maternal Deaths
- Machine Learning-Enabled Blood Test Predicts Immunotherapy Response in Lymphoma Patients
- Post-Treatment Blood Test Could Inform Future Cancer Therapy Decisions
- Cerebrospinal Fluid Test Predicts Dangerous Side Effect of Cancer Treatment
- New Test Measures Preterm Infant Immunity Using Only Two Drops of Blood
- Simple Blood Test Could Help Choose Better Treatments for Patients with Recurrent Endometrial Cancer
- Breakthrough Diagnostic Technology Identifies Bacterial Infections with Almost 100% Accuracy within Three Hours
- Innovative ID/AST System to Help Diagnose Infectious Diseases and Combat AMR
- Gastrointestinal Panel Delivers Rapid Detection of Five Common Bacterial Pathogens for Outpatient Use
- Rapid PCR Testing in ICU Improves Antibiotic Stewardship
- Unique Genetic Signature Predicts Drug Resistance in Bacteria
- Smartphones Could Diagnose Diseases Using Infrared Scans
- Novel Sensor Technology to Enable Early Diagnoses of Metabolic and Cardiovascular Disorders
- 3D Printing Breakthrough Enables Large Scale Development of Tiny Microfluidic Devices
- POC Paper-Based Sensor Platform to Transform Cardiac Diagnostics
- Study Explores Impact of POC Testing on Future of Diagnostics
- New Collaboration to Advance Microbial Identification for Infectious Disease Diagnostics
- Tecan Acquires ELISA Immunoassay Assets from Revvity's Cisbio Bioassays
- Leica Biosystems and Bio-Techne Expand Spatial Multiomic Collaboration
- Philips and Ibex Expand Partnership to Enhance AI-Enabled Pathology Workflows
- Grifols and Inpeco Partner to Deliver Transfusion Medicine ‘Lab of The Future’
- Gene Panel Predicts Disease Progession for Patients with B-cell Lymphoma
- New Method Simplifies Preparation of Tumor Genomic DNA Libraries
- New Tool Developed for Diagnosis of Chronic HBV Infection
- Panel of Genetic Loci Accurately Predicts Risk of Developing Gout
- Disrupted TGFB Signaling Linked to Increased Cancer-Related Bacteria
- New Laser-Based Method to Accelerate Cancer Diagnosis
- New AI Model Predicts Gene Variants’ Effects on Specific Diseases
- Powerful AI Tool Diagnoses Coeliac Disease from Biopsy Images with Over 97% Accuracy
- Pre-Analytical Conditions Influence Cell-Free MicroRNA Stability in Blood Plasma Samples
- 3D Cell Culture System Could Revolutionize Cancer Diagnostics