Expo
view channel
view channel
view channel
view channel
view channel
view channel
view channel
view channel
view channel
Clinical Chem.Molecular DiagnosticsHematology
MicrobiologyPathologyTechnologyIndustry
Events
- Paper-Based Device Boosts HIV Test Accuracy from Dried Blood Samples
- AI-Powered Raman Spectroscopy Method Enables Rapid Drug Detection in Blood
- Novel LC-MS/MS Assay Detects Low Creatinine in Sweat and Saliva
- Biosensing Technology Breakthrough Paves Way for New Methods of Early Disease Detection
- New Saliva Test Rapidly Identifies Paracetamol Overdose
- Simple Urine Test Could Help Patients Avoid Invasive Scans for Kidney Cancer
- New Bowel Cancer Blood Test to Improve Early Detection
- New Method Rapidly Diagnoses CVD Risk Via Molecular Blood Screening
- Refined Test Improves Parkinson’s Disease Diagnosis
- Blood Test Shows Promise for Early Detection of Dementia
- Non-Invasive Prenatal Test for Fetal RhD Status Demonstrates 100% Accuracy
- WBC Count Could Predict Severity of COVID-19 Symptoms
- New Platelet Counting Technology to Help Labs Prevent Diagnosis Errors
- Streamlined Approach to Testing for Heparin-Induced Thrombocytopenia Improves Diagnostic Accuracy
- POC Hemostasis System Could Help Prevent Maternal Deaths
- Cerebrospinal Fluid Test Predicts Dangerous Side Effect of Cancer Treatment
- New Test Measures Preterm Infant Immunity Using Only Two Drops of Blood
- Simple Blood Test Could Help Choose Better Treatments for Patients with Recurrent Endometrial Cancer
- Novel Analytical Method Tracks Progression of Autoimmune Diseases
- 3D Bioprinted Gastric Cancer Model Uses Patient-Derived Tissue Fragments to Predict Drug Response
- Gastrointestinal Panel Delivers Rapid Detection of Five Common Bacterial Pathogens for Outpatient Use
- Rapid PCR Testing in ICU Improves Antibiotic Stewardship
- Unique Genetic Signature Predicts Drug Resistance in Bacteria
- Unique Barcoding System Tracks Pneumonia-Causing Bacteria as They Infect Blood Stream
- Rapid Sepsis Diagnostic Test Demonstrates Improved Patient Care and Cost Savings in Hospital Application
- 3D Printing Breakthrough Enables Large Scale Development of Tiny Microfluidic Devices
- POC Paper-Based Sensor Platform to Transform Cardiac Diagnostics
- Study Explores Impact of POC Testing on Future of Diagnostics
- Low-Cost, Fast Response Sensor Enables Early and Accurate Detection of Lung Cancer
- Nanotechnology For Cervical Cancer Diagnosis Could Replace Invasive Pap Smears
- Grifols and Inpeco Partner to Deliver Transfusion Medicine ‘Lab of The Future’
- Research Collaboration to Advance AI-Enhanced, Real-Time Optical Imaging in Lung Cancer Biopsy
- CACLP 2025 Unites Global Innovators in IVD Industry
- Bio-Rad to Acquire Digital PCR Developer Stilla Technologies
- ABL Signs Know-How License and Transfer Agreement for Siemens’ Fast Track Diagnostics PCR Portfolio
- Gene Panel Predicts Disease Progession for Patients with B-cell Lymphoma
- New Method Simplifies Preparation of Tumor Genomic DNA Libraries
- New Tool Developed for Diagnosis of Chronic HBV Infection
- Panel of Genetic Loci Accurately Predicts Risk of Developing Gout
- Disrupted TGFB Signaling Linked to Increased Cancer-Related Bacteria
- Pre-Analytical Conditions Influence Cell-Free MicroRNA Stability in Blood Plasma Samples
- 3D Cell Culture System Could Revolutionize Cancer Diagnostics
- Painless Technique Measures Glucose Concentrations in Solution and Tissue Via Sound Waves
- Skin-Based Test to Improve Diagnosis of Rare, Debilitating Neurodegenerative Disease
- Serum Uromodulin Could Indicate Acute Kidney Injury in COVID-19 Patients
Expo
view channel
view channel
view channel
view channel
view channel
view channel
view channel
view channel
view channel
Clinical Chem.Molecular DiagnosticsHematology
MicrobiologyPathologyTechnologyIndustry
Events
Advertise with Us
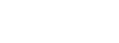
- Paper-Based Device Boosts HIV Test Accuracy from Dried Blood Samples
- AI-Powered Raman Spectroscopy Method Enables Rapid Drug Detection in Blood
- Novel LC-MS/MS Assay Detects Low Creatinine in Sweat and Saliva
- Biosensing Technology Breakthrough Paves Way for New Methods of Early Disease Detection
- New Saliva Test Rapidly Identifies Paracetamol Overdose
- Simple Urine Test Could Help Patients Avoid Invasive Scans for Kidney Cancer
- New Bowel Cancer Blood Test to Improve Early Detection
- New Method Rapidly Diagnoses CVD Risk Via Molecular Blood Screening
- Refined Test Improves Parkinson’s Disease Diagnosis
- Blood Test Shows Promise for Early Detection of Dementia
- Non-Invasive Prenatal Test for Fetal RhD Status Demonstrates 100% Accuracy
- WBC Count Could Predict Severity of COVID-19 Symptoms
- New Platelet Counting Technology to Help Labs Prevent Diagnosis Errors
- Streamlined Approach to Testing for Heparin-Induced Thrombocytopenia Improves Diagnostic Accuracy
- POC Hemostasis System Could Help Prevent Maternal Deaths
- Cerebrospinal Fluid Test Predicts Dangerous Side Effect of Cancer Treatment
- New Test Measures Preterm Infant Immunity Using Only Two Drops of Blood
- Simple Blood Test Could Help Choose Better Treatments for Patients with Recurrent Endometrial Cancer
- Novel Analytical Method Tracks Progression of Autoimmune Diseases
- 3D Bioprinted Gastric Cancer Model Uses Patient-Derived Tissue Fragments to Predict Drug Response
- Gastrointestinal Panel Delivers Rapid Detection of Five Common Bacterial Pathogens for Outpatient Use
- Rapid PCR Testing in ICU Improves Antibiotic Stewardship
- Unique Genetic Signature Predicts Drug Resistance in Bacteria
- Unique Barcoding System Tracks Pneumonia-Causing Bacteria as They Infect Blood Stream
- Rapid Sepsis Diagnostic Test Demonstrates Improved Patient Care and Cost Savings in Hospital Application
- 3D Printing Breakthrough Enables Large Scale Development of Tiny Microfluidic Devices
- POC Paper-Based Sensor Platform to Transform Cardiac Diagnostics
- Study Explores Impact of POC Testing on Future of Diagnostics
- Low-Cost, Fast Response Sensor Enables Early and Accurate Detection of Lung Cancer
- Nanotechnology For Cervical Cancer Diagnosis Could Replace Invasive Pap Smears
- Grifols and Inpeco Partner to Deliver Transfusion Medicine ‘Lab of The Future’
- Research Collaboration to Advance AI-Enhanced, Real-Time Optical Imaging in Lung Cancer Biopsy
- CACLP 2025 Unites Global Innovators in IVD Industry
- Bio-Rad to Acquire Digital PCR Developer Stilla Technologies
- ABL Signs Know-How License and Transfer Agreement for Siemens’ Fast Track Diagnostics PCR Portfolio
- Gene Panel Predicts Disease Progession for Patients with B-cell Lymphoma
- New Method Simplifies Preparation of Tumor Genomic DNA Libraries
- New Tool Developed for Diagnosis of Chronic HBV Infection
- Panel of Genetic Loci Accurately Predicts Risk of Developing Gout
- Disrupted TGFB Signaling Linked to Increased Cancer-Related Bacteria
- Pre-Analytical Conditions Influence Cell-Free MicroRNA Stability in Blood Plasma Samples
- 3D Cell Culture System Could Revolutionize Cancer Diagnostics
- Painless Technique Measures Glucose Concentrations in Solution and Tissue Via Sound Waves
- Skin-Based Test to Improve Diagnosis of Rare, Debilitating Neurodegenerative Disease
- Serum Uromodulin Could Indicate Acute Kidney Injury in COVID-19 Patients