Expo
MEDLAB
view channel
view channel
view channel
view channel
view channel
view channel
view channel
view channel
view channel
Clinical Chem.Molecular DiagnosticsHematology
MicrobiologyPathologyTechnologyIndustry
Events
- Novel LC-MS/MS Assay Detects Low Creatinine in Sweat and Saliva
- Biosensing Technology Breakthrough Paves Way for New Methods of Early Disease Detection
- New Saliva Test Rapidly Identifies Paracetamol Overdose
- POC Saliva Testing Device Predicts Heart Failure in 15 Minutes
- Screening Tool Detects Multiple Health Conditions from Single Blood Drop
- Breakthrough Technology Represents Leap Forward in Next-Generation Sequencing
- New Lipid Blood Test Identifies Children at Risk of Diabetes
- ‘Super Test’ for Prostate Cancer to Revolutionize Screening and Diagnosis
- Noninvasive Urinary DNA Methylation Test Accurately Diagnoses Bladder Cancer
- New Blood Test Improves Diagnosis of Alzheimer’s Disease
- New Platelet Counting Technology to Help Labs Prevent Diagnosis Errors
- Streamlined Approach to Testing for Heparin-Induced Thrombocytopenia Improves Diagnostic Accuracy
- POC Hemostasis System Could Help Prevent Maternal Deaths
- New Test Assesses Oxygen Delivering Ability of Red Blood Cells by Measuring Their Shape
- Personalized CBC Testing Could Help Diagnose Early-Stage Diseases in Healthy Individuals
- 3D Bioprinted Gastric Cancer Model Uses Patient-Derived Tissue Fragments to Predict Drug Response
- Cutting-Edge Microscopy Technology Enables Tailored Rheumatology Therapies
- New Discovery in Blood Immune Cells Paves Way for Parkinson's Disease Diagnostic Test
- AI Tool Uses Routine Blood Tests to Predict Immunotherapy Response for Various Cancers
- Blood Test Can Predict How Long Vaccine Immunity Will Last
- Rapid Diagnostic System to Detect Neonatal Sepsis Within Hours
- Novel Test to Diagnose Bacterial Pneumonia Directly from Whole Blood
- Interferon-γ Release Assay Effective in Patients with COPD Complicated with Pulmonary Tuberculosis
- New Point of Care Tests to Help Reduce Overuse of Antibiotics
- 30-Minute Sepsis Test Differentiates Bacterial Infections, Viral Infections, and Noninfectious Disease
- Study Explores Impact of POC Testing on Future of Diagnostics
- Low-Cost, Fast Response Sensor Enables Early and Accurate Detection of Lung Cancer
- Nanotechnology For Cervical Cancer Diagnosis Could Replace Invasive Pap Smears
- Lab-On-Chip Platform to Expedite Cancer Diagnoses
- Biosensing Platform Simultaneously Detects Vitamin C and SARS-CoV-2
- ABL Signs Know-How License and Transfer Agreement for Siemens’ Fast Track Diagnostics PCR Portfolio
- Becton Dickinson to Spin Out Biosciences and Diagnostic Solutions Business
- New Partnership Revolutionizes Analyses of Biological Samples
- Medlab Middle East Looks to The Future of Laboratories
- Medix Biochemica Acquires German Immunoassay Solutions Developer Candor Bioscience
- Gene Panel Predicts Disease Progession for Patients with B-cell Lymphoma
- New Method Simplifies Preparation of Tumor Genomic DNA Libraries
- New Tool Developed for Diagnosis of Chronic HBV Infection
- Panel of Genetic Loci Accurately Predicts Risk of Developing Gout
- Disrupted TGFB Signaling Linked to Increased Cancer-Related Bacteria
- AI-Driven Tool to Accelerate Cancer Diagnosis
- Revolutionary AI Tool Transforms Disease Visualization
- Novel Dataset of Plasma Cells to Aid Diagnosis of Multiple Myeloma
- New Metabolite Detection Method Using DNA Sequencing Could Transform Diagnostics
- AI-Powered Pathology Solutions Accurately Predict Outcomes for HER2-Targeted Therapy in Metastatic CRC
Expo
MEDLAB
view channel
view channel
view channel
view channel
view channel
view channel
view channel
view channel
view channel
view channel
view channel
view channel
view channel
view channel
view channel
view channel
view channel
Clinical Chem.Molecular DiagnosticsHematology
MicrobiologyPathologyTechnologyIndustry
Events
Advertise with Us
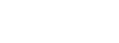
- Novel LC-MS/MS Assay Detects Low Creatinine in Sweat and Saliva
- Biosensing Technology Breakthrough Paves Way for New Methods of Early Disease Detection
- New Saliva Test Rapidly Identifies Paracetamol Overdose
- POC Saliva Testing Device Predicts Heart Failure in 15 Minutes
- Screening Tool Detects Multiple Health Conditions from Single Blood Drop
- Breakthrough Technology Represents Leap Forward in Next-Generation Sequencing
- New Lipid Blood Test Identifies Children at Risk of Diabetes
- ‘Super Test’ for Prostate Cancer to Revolutionize Screening and Diagnosis
- Noninvasive Urinary DNA Methylation Test Accurately Diagnoses Bladder Cancer
- New Blood Test Improves Diagnosis of Alzheimer’s Disease
- New Platelet Counting Technology to Help Labs Prevent Diagnosis Errors
- Streamlined Approach to Testing for Heparin-Induced Thrombocytopenia Improves Diagnostic Accuracy
- POC Hemostasis System Could Help Prevent Maternal Deaths
- New Test Assesses Oxygen Delivering Ability of Red Blood Cells by Measuring Their Shape
- Personalized CBC Testing Could Help Diagnose Early-Stage Diseases in Healthy Individuals
- 3D Bioprinted Gastric Cancer Model Uses Patient-Derived Tissue Fragments to Predict Drug Response
- Cutting-Edge Microscopy Technology Enables Tailored Rheumatology Therapies
- New Discovery in Blood Immune Cells Paves Way for Parkinson's Disease Diagnostic Test
- AI Tool Uses Routine Blood Tests to Predict Immunotherapy Response for Various Cancers
- Blood Test Can Predict How Long Vaccine Immunity Will Last
- Rapid Diagnostic System to Detect Neonatal Sepsis Within Hours
- Novel Test to Diagnose Bacterial Pneumonia Directly from Whole Blood
- Interferon-γ Release Assay Effective in Patients with COPD Complicated with Pulmonary Tuberculosis
- New Point of Care Tests to Help Reduce Overuse of Antibiotics
- 30-Minute Sepsis Test Differentiates Bacterial Infections, Viral Infections, and Noninfectious Disease
- Study Explores Impact of POC Testing on Future of Diagnostics
- Low-Cost, Fast Response Sensor Enables Early and Accurate Detection of Lung Cancer
- Nanotechnology For Cervical Cancer Diagnosis Could Replace Invasive Pap Smears
- Lab-On-Chip Platform to Expedite Cancer Diagnoses
- Biosensing Platform Simultaneously Detects Vitamin C and SARS-CoV-2
- ABL Signs Know-How License and Transfer Agreement for Siemens’ Fast Track Diagnostics PCR Portfolio
- Becton Dickinson to Spin Out Biosciences and Diagnostic Solutions Business
- New Partnership Revolutionizes Analyses of Biological Samples
- Medlab Middle East Looks to The Future of Laboratories
- Medix Biochemica Acquires German Immunoassay Solutions Developer Candor Bioscience
- MEDLAB Europe 2018 Presents Latest Technologies and Developments
- Siemens Healthineers Showcases Advanced Diagnostics Analyzers
- BIOBASE Displays Range Of Laboratory & Medical Analyzers
- Fujifilm Showcases DRI-CHEM NX700 Dry Chemistry Analyzer
- MedLab Middle East Continues as World's Largest Attended Laboratory Exhibition and Conference
- Telstar Promotes Latest Generation of Freeze Dryers in Dubai
- Siemens Healthineers Showcases In-Vitro Diagnostics Products at MedLab 2019
- Randox Promotes Evidence MultiSTAT Automated Benchtop Immunoassay Analyzer
- Mindray Displays New Generation Benchtop Cellular Analysis Line
- HORIBA Medical Shows Latest Yumizen G Range at Lab Trade Show
- Gene Panel Predicts Disease Progession for Patients with B-cell Lymphoma
- New Method Simplifies Preparation of Tumor Genomic DNA Libraries
- New Tool Developed for Diagnosis of Chronic HBV Infection
- Panel of Genetic Loci Accurately Predicts Risk of Developing Gout
- Disrupted TGFB Signaling Linked to Increased Cancer-Related Bacteria
- DAS Srl Exhibits Range of Fully Automated Systems at MEDLAB Middle East 2020
- GeneMatrix Promotes Sex and Respiratory Infection Testing Products at MEDLAB
- Mindray Showcases Comprehensive IVD Solutions Portfolio at MEDLAB Middle East 2020
- New Technology that Detects MRSA in 15 Minutes Introduced at MEDLAB Middle East 2020
- Vircell Exhibits Its New VirClia Lotus and qSPEED-OLIGO Systems at MEDLAB
- MP Biomedicals Exhibits Diagnostics Kits and Sample Prep Solutions at MEDLAB Middle East 2021
- BD Demonstrates Latest Medtech Innovations at MEDLAB Middle East 2021
- Diagnostica Stago Highlights Max Generation Family of Coagulation Analyzers at MEDLAB Middle East 2021
- Randox Showcases Latest Product Portfolio and Services at MEDLAB Middle East 2021
- Horiba Medical Exhibits New Generation of Automated Hematology Analyzers at MEDLAB Middle East 2021
- PerkinElmer Exhibits Automated Nucleic Acid Extraction Solutions at MEDLAB Middle East
- Abbott Displays Its Life-Changing POC Tests and Diagnostic Tools at MEDLAB Middle East
- Diagast Presents Latest Immunohaematology Solutions at MEDLAB Middle East
- Siemens Brings Its Latest Innovations in Laboratory Diagnostics to MEDLAB Middle East 2022
- Bioperfectus Showcases Its Molecular Diagnostic Products for Infectious Diseases at MEDLAB 2022 Online
- SNIBE Brings Latest Innovations in Immunoassay, Clinical Biochemistry and Molecular Diagnostics to Medlab Middle East 2023
- Sansure Exhibits Portable Molecular Workstation at Medlab Middle East 2023
- QIAGEN Demonstrates Its Revolutionary Molecular Diagnostic Solution at Medlab Middle East 2023
- QuidelOrtho Demonstrates Automated, Sample-To-Result, Multiplex RT-PCR Testing Platform at Medlab Middle East 2023
- Erba Introduces XL Range of Fully Automated Clinical Chemistry Systems at Medlab Middle East 2023
- 77 Elektronika Showcases Latest Developments in Urinalysis
- BioVendor Group Unveils Latest Innovations in Immunodiagnostics and Molecular Diagnostics
- Sansure Presents Future of Pathogen Identification and Disease Diagnosis
- Spinreact Presents Latest Developments in IVD
- Alifax Demonstrates Automated Solutions to Improve Performance in Laboratory Settings
- BIOBASE Showcases Comprehensive Range of Laboratory Solutions
- Dymind Presents Advanced Automatic Hematology Analysis Line
- BioPerfectus Presents Latest Solutions for Women's Health and Infectious Disease Diagnosis
- YHLO Unveils Total Laboratory Automation System for Elevated Laboratory Efficiency
- Vazyme Showcases Cutting-Edge Microfluidic Solutions for Precise and Efficient Laboratory Workflows
- AI-Driven Tool to Accelerate Cancer Diagnosis
- Revolutionary AI Tool Transforms Disease Visualization
- Novel Dataset of Plasma Cells to Aid Diagnosis of Multiple Myeloma
- New Metabolite Detection Method Using DNA Sequencing Could Transform Diagnostics
- AI-Powered Pathology Solutions Accurately Predict Outcomes for HER2-Targeted Therapy in Metastatic CRC